Keynote Speeches
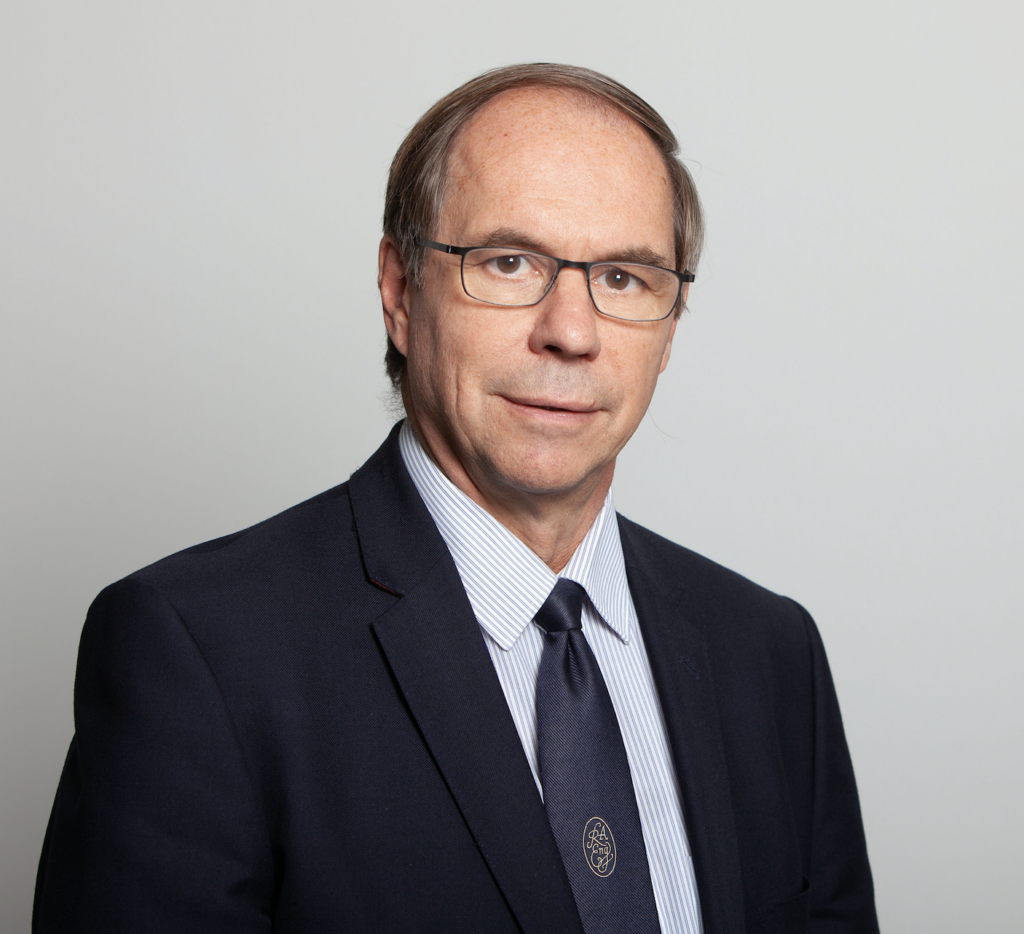
Apps, wearables and machine learning for the COVID-19 pandemic
Since the beginning of the pandemic in March 2020, work at the Institute of Biomedical Engineering in the University of Oxford and at Sensyne Health, a Clinical AI company on the Oxford Science Park, has focused on improving outcomes for patients infected by the SARS-CoV-2 virus, or for individuals at risk because of possible infection by the virus. This has included the use of a remote monitoring app for pregnant women with high blood pressure and at risk of pre-eclampsia, to eliminate the need for them to attend hospital clinics fortnightly for blood pressure monitoring. In the hospital, continuous vital-sign monitoring using wearables has enabled the identification of the rapid desaturation caused by the SARS-CoV-2 virus, and intervention with corrective treatment at the earliest opportunity (e.g. via additional oxygen therapy). Use of the system decreases the amount of contact between the patient and nursing staff, reducing the risk of COVID-19 transmission. Finally, the reliability of lateral flow tests to detect infection in asymptomatic individuals has been improved by the design of an app to record the test result by taking a photo of the device and using machine learning algorithms for the automatic classification of the result.
Speaker
Professor Lionel Tarassenko CBE FREng FMedSci is a world-leading expert in the application of signal processing and machine learning to healthcare, with a strong track record in translation to clinical medicine. His work has had a major impact on the identification of deterioration in acute care and on the management of chronic disease.
Professor Tarassenko received the BA in Engineering Science in 1978 and the DPhil in medical electronics in 1985, both from the University of Oxford. After a period in industry, he was appointed University Lecturer in 1988. He was elected to the Chair of Electrical Engineering, also at the University of Oxford, in 1997. He was the driving force behind the creation of the Institute of Biomedical Engineering which he directed from its opening in April 2008 to October 2012. Under his leadership, the Institute grew from 110 to 220 academic researchers, and it was awarded a Queen’s Anniversary Prize for Higher Education in 2015 for “new collaborations between engineering and medicine delivering benefit to patients”.
Professor Tarassenko was elected to a Fellowship of the Royal Academy of Engineering in 2000, and to a Fellowship of the Academy of Medical Sciences in 2013. He is the author of 230 journal papers, 210 conference papers, 3 books and 32 granted patents. He was the Head of the Department of Engineering Science (Dean of Engineering) from 2014 to 2019, and is now the Founding President of Reuben College, the University of Oxford’s newest college. He has founded four University spin-out companies and is the R&D Director of Sensyne Health, an AIM-listed company.

The Transformational Journey and Future of Surgical Robotics
The use of surgical robotics has seen tremendous growth over the last 20 years and now plays an important role in the field of surgery. These systems allow more procedures to be performed using minimally invasive techniques and improve outcomes over open surgery by reducing trauma and shortening recovery times for patients. The exciting reality is that this is just the beginning of the transformational power of this technology. The future of this field lies in the combination of robotics with advanced visualization, navigation, and data analytics. This presentation will give an overview of Medtronic’s new Hugo Surgical Robotic System and discuss how it is a platform for growth in these areas to integrate new technology and enable advanced procedures. The presentation will also explore ways that industry and academia can better partner to realize the full potential of surgical robotics by leveraging our strengths and working together.
Speaker
Bill Peine, Ph.D. is the Senior Director of System Architecture at Medtronic’s Surgical Robotics Unit developing the Hugo Robotic System for teleoperated minimally invasive surgery. Before joining the company in 2013, Bill was at T2 BioSystems working as a technical lead to develop the T2Dx, a fluid diagnostic system incorporating nuclear magnetic resonance for detecting molecular targets. He has also been involved with several medical device startups, including Cambridge Endoscopic Devices developing handheld articulating laparoscopic instruments and EndoVia Medical developing a low-cost teleoperated surgical robot. In addition to his industrial experience, Bill was a tenure track Professor of Mechanical Engineering at Purdue University researching novel surgical robotic technology and algorithms for image-guided surgery. He was also a founder of Pressure Profile Systems producing high sensitivity tactile sensors and systems for industrial and academic applications. Bill earned his Ph.D. in Engineering Sciences from Harvard University and B.S. in Electrical Engineering from Purdue University and is the author of over 80 patents and peer reviewed publications.
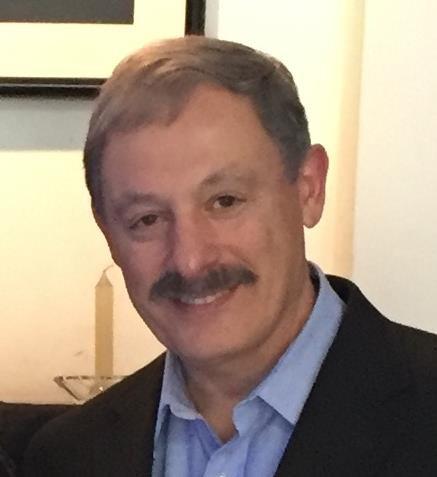
Blockchain for Transactive Energy Management in Renewable Energy Systems
This presentation offers a vision and analyzes a scheme developed for microgrids that utilizes blockchain technologies to optimize transactive operations in power distribution systems. Technological and socioeconomic developments in distributed energy resources have promoted the use of renewable energy in microgrids as a promising alternative to the traditional use of thermal energy for electricity delivery in a bulk power grid. Microgrids are small-scale self-controllable power systems that interconnect on-site generation resources and loads for striking a local balance of energy production and consumption. Geographically-close microgrids are networked to broaden the merits of microgrids and eventually refine electricity service provisions across the power distribution system. Transactive energy, as the cross-section of technological, political and economic innovations, opens up the door to peer-to-peer autonomous electricity retail markets together with new business and operation models for power generation, delivery, and consumption. Through active participation in the decentralized transactive energy management process, networked microgrids collectively provide additional opportunities for improving the operational performance of power distribution systems. As networked microgrids are intrinsically cyber-physical systems that may unintentionally expose cybersecurity vulnerabilities to potential disruptive agents, blockchain is considered as an option that provides a transformative solution to address the cybersecurity and mutual-trust concerns through the application of cryptography and the execution of smart contracts. Accordingly, networked microgrids will interact in an automatic, credible and auditable manner for maintaining a greater degree of efficiency, reliability, resilience, and sustainability in electricity services offered to local communities. We present several practical examples and conclude that blockchain technologies embedded in transactive energy will play a significant role in the evolution of traditional power distribution systems to active and smart distribution networks.
Speaker
Dr. Mohammad Shahidehpour is a University Distinguished Professor, Bodine Chair Professor of Electrical and Computer Engineering, and Director of the Robert W. Galvin Center for Electricity Innovation at Illinois Institute of Technology (IIT). His project on Perfect Power Systems has converted the entire IIT Campus to an islandable microgrid. Dr. Shahidehpour was the recipient of several technical awards including of the IEEE Burke Hayes Award for his research on hydrokinetics, IEEE/PES Outstanding Power Engineering Educator Award, IEEE/PES Ramakumar Family Renewable Energy Excellence Award, IEEE/PES Douglas M. Staszesky Distribution Automation Award, and the Edison Electric Institute’s Power Engineering Educator Award. He has co-authored 6 books and 750 technical papers on electric power system operation and planning, and served as the founding Editor-in-Chief of the IEEE Transactions on Smart Grid. Dr. Shahidehpour is the recipient of the 2009 honorary doctorate from the Polytechnic University of Bucharest. He is a Fellow of IEEE, Fellow of the American Association for the Advancement of Science (AAAS), Fellow of the National Academy of Inventors (NAI), and an elected member of the US National Academy of Engineering (NAE). He is also listed as a highly cited researcher on the Web of Science (ranked in the top 1% by citations demonstrating significant influence among his peers).
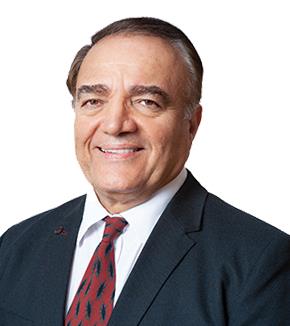
The Enabling Technologies for Exploration of Space Missions
For space, technology carries a specific meaning: the means to enable exciting new missions. We have achieved many new capabilities by leveraging technologies that have seen rapid advances for mission applications. For example, the advances in hi-speed rad-hard processors is enabling autonomous spacecraft missions. Future ground-breaking discoveries, as well as NASA’s role in that future, will depend on autonomous systems, that use sensing, perception, reasoning, and decision-making, with little to no human intervention. The advantages of rapid 3D printing have been pushed to an additional fourth “dimension,” namely by constructing space systems whose functions evolve over the course of the mission. The use of the smart materials has now made it possible to develop gears that no longer require lubrication and will perform in extreme environment for a long time. Other examples include miniaturization of instruments and systems now flying in JPL’s planetary CubeSats, new robotic systems that can crawl on a wall, and the swarms of thousands of tiny autonomous spacecraft, as well as flying a helicopter in Mars atmosphere. This presentation will provide an overview of the emerging space missions and the breakthrough technologies that will enable these missions over the next two decades with emphasis on challenges in operation of autonomous systems.
Speaker
Dr. Hadaegh is the Jet Propulsion Laboratory’s Chief Technologist. He joined JPL in 1984 after receiving his Ph.D. in Electrical Engineering from the University of Southern California. For over two decades he led the Guidance and Control (G&C) Analysis Group for research in guidance, estimation, and control theory and for the development of algorithms and software for planetary science and astrophysics missions. He has been Principal Investigator for several space technology demonstrations and has led technology developments for spacecraft formation flying, autonomous rendezvous and docking for NASA missions and the Department of Defense programs.
Hadaegh is a JPL Fellow, Senior Research Scientist, and a Principal Engineer. He is a Fellow of the Institute of Electronics and Electrical Engineers (IEEE) and Fellow of the American Institute of Aeronautics and Astronautics (AIAA). He has received numerous awards including NASA’s Exceptional Service and Exceptional Achievement Medals, the AIAA ‘s 2021 Mechanics and Control of Flight Award, and JPL’s Award of Excellence for “Flight Validation of Autonomous Rendezvous in Earth Orbit.”
His research interests include optimal estimation and control of spacecraft systems. He has published extensively on mathematical modeling of uncertain systems, parameter identifiability of dynamical systems, identification and control of large space structures, and autonomous control of distributed spacecraft.
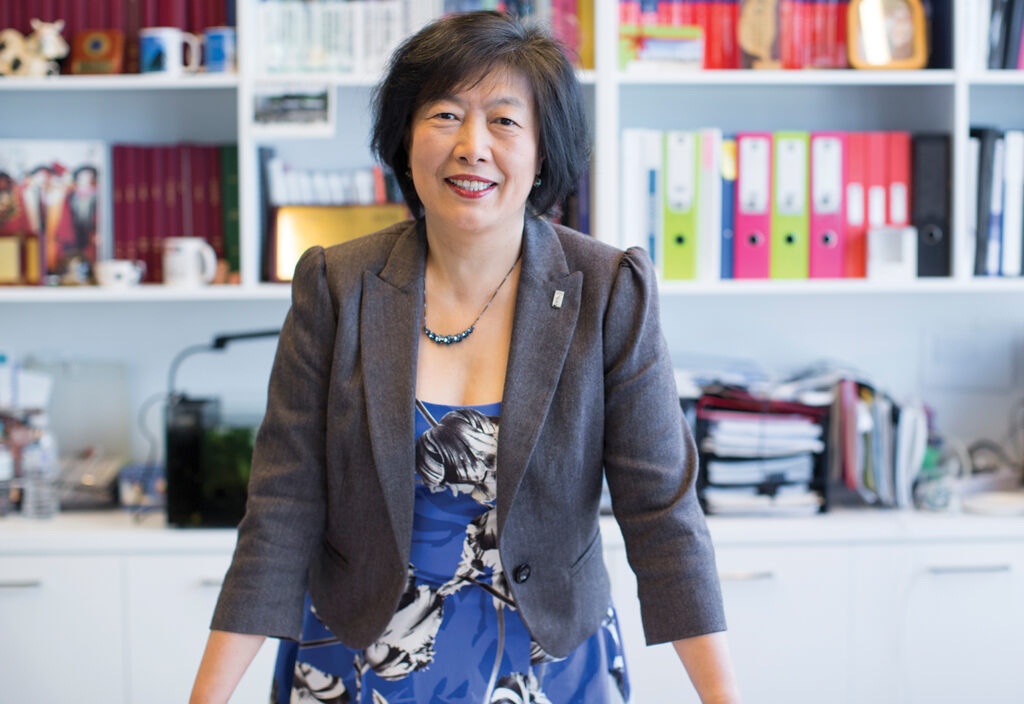
Machine Learning for Decision Support in Complex Environments
This talk will present how machine learning can innovatively and effectively learn from data to support data-driven decision-making in uncertain and dynamic situations. Fuzzy Transfer Learning theories, methodologies and algorithms will be presented that can transfer knowledge learnt from one or more source domains to target domains. Knowledge transfer is achieved by building latent spaces, fuzzy mapping functions and self-training to overcome tremendous uncertainties in data, learning processes and decision outputs (classification and regression). This talk will also present Concept Drift theories, methodologies and algorithms to show how drift learning can handle ever-changing dynamic data stream environments with unpredictable stream pattern drifts through developing effective and accurate detection and adaptation methods. These new developments enable machine learning to enhance data-driven prediction and decision support systems in uncertain and dynamic environments
Speaker
Distinguished Professor Jie Lu is an internationally renowned scientist specializing in fuzzy transfer learning, concept drift, decision support systems, and recommender systems. She is an IEEE Fellow, IFSA Fellow and Australian Laureate Fellow and Director of the Australian Artificial Intelligence Institute (AAII) which has over 250 staff and students and the Associate Dean (Research Excellence) in the Faculty of Engineering and Information Technology at the University of Technology Sydney (UTS). Distinguished Professor Lu has published six research books and over 400 papers in Artificial Intelligence, IEEE Transactions on Fuzzy Systems, IEEE Transactions on Neural Networks and Learning Systems, IEEE Transactions on Cybernetics, IEEE Transactions on SMC: Systems, and other leading journals and conference proceedings, including ICML, IJCAI, AAAI, ICDM. She has won an ARC Australian Laureate Fellowship and ten ARC Discovery Projects, led many industry research projects and supervised 46 PhD students to completion. Within the scientific community, she serves as Editor-In-Chief for Knowledge-Based Systems (Elsevier), Editor-In-Chief for the International Journal of Computational Intelligence Systems and has delivered more than 25 keynote speeches at international conferences and chaired 15 international conferences. Distinguished Professor Lu is the recipient of the UTS Medal for Research and Teaching Integration (2010), the UTS Medal for Research Excellence (2019), the Computer Journal Wilkes Award (2018), the IEEE Transactions on Fuzzy Systems Outstanding Paper Award (2019) and the Australian Most Innovative Engineer Award (2019).
.
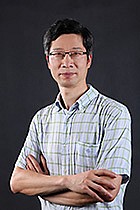
Ensemble Approaches to Class Imbalance Learning
Many real world classification problems have highly imbalanced and skew data distributions. In fault
diagnosis and condition monitoring for example, there are ample data for the normal class, yet data for faults are always very limited and costly to obtain. It is often a challenge to increase the performance of a classifier on the minority classes without sacrificing the performance on the majority classes. This talk discusses some of the techniques and algorithms that have been developed for class imbalance learning, especially through ensemble learning. First, the motivations behind ensemble learning are introduced and the importance of diversity highlighted. Second, some of the challenges of multi-class imbalance learning and potential solutions are presented. What might have worked well for the binary case do not work for multiple classes anymore, especially when the number of classes increases. Third, online class imbalance learning will be discussed, which can be seen as a combination of online learning and class imbalance learning. Online class imbalance learning poses new research challenges that still have not been well understood., let alone solved, especially for imbalanced data streams with concept drift. Fourth, the natural fit of multi-objective learning to class imbalance learning is pointed out. The relationship between multi-objective learning and ensemble learning will be discussed. Finally, future research directions will be given.
Speaker
Xin Yao is a Chair Professor of Computer Science at the Southern University of Science and Technology, Shenzhen, China, and a part-time professor at the University of Birmingham, UK. His major research interests include evolutionary computation, ensemble learning and search-based software engineering. He is an IEEE, a former (2014-15) president of IEEE Computational Intelligence Society and a former (20003-08) Editor-in-Chief of IEEE Transactions on Evolutionary Computation. His work won the 2001 IEEE Donald G. Fink Prize Paper Award, 2010, 2016 and 2017 IEEE Transactions on Evolutionary Computation Outstanding Paper Awards, 2010 BT Gordon Radley Award for Best Author of Innovation (Finalist), 2011 IEEE Transactions on Neural Networks Outstanding Paper Award, and many other best paper awards. He received a Royal Society Wolfson Research Merit Award in 2012, the IEEE CIS Evolutionary Computation Pioneer Award in 2013, and the 2020 IEEE Frank Rosenblatt Award.

Realising Autonomy in Army
The Australian Army published its Robotic and Autonomous Systems Strategy in 2018; since then it has been implementing it. Bringing emerging technology into reality, and the exploring the potential impact for a future Army is challenging. In the presentation, we will explore the strategy and through case studies will explore opportunities and challenges of realising autonomy for the Army.
Speaker
Colonel Robin Smith is the inaugural Director of the Army’s Robotic & Autonomous Systems Implementation & Coordination Office (RICO) within Future Land Warfare Branch of the Australian Army HQ. RICO leads concept development around emerging and disruptive technology, including autonomy and AI, Quantum Technologies and alternative power and energy. He has overseen Army’s exploration of autonomous systems since 2017 after he authored the Australian Army Robotic and Autonomous Systems Strategy.
He is a logistician by background and served for over 30 years in the British Army before transferring to the Australian Army. He has commanded at all levels up to and including Regimental level and been deployed operationally a number of times including Afghanistan multiple times, Sierra Leone and supported other deployments for which has was awarded the OBE in 2013. He has a background in Electronic Engineering and has fulfilled a number of roles in the Joint logistics domain at the tactical and operational levels